Introduction
Imagine you’re trying to figure out the value of an option, which is a type of financial instrument. One important thing to consider is how the underlying asset changes over time. We call this change a stochastic process, which basically means they evolve in a random manner. Now, some financial instruments have a special feature called limited liability, which means their values can’t go below zero. However, they can be worth zero if things don’t go well (think about a stock, the GameStop Corp stock for instance, NYSE: GME). Back in 1900, a French mathematician named Louis Bachelier suggested using a probability distribution called the normal distribution (i.e. Gaussian) to model these changes. He was right, but his work was recognized as the first mathematically rigorous option valuation model more than 50 years later when the Black-Scholes-Merton Model won the Nobel Prize: the formulas in their winning papers were incredibly close to Bachelier’s assumptions. In this article, we will come back to origins and then we will try to assess the validity of Bachelier’s Speculation Theory in modern markets and explore the key factors that impact its applicability.
Table of Contents
- Introduction
- The Theory of Speculation
- From Bachelier’s stochasticity to alternative approaches
- Bachelier’s Speculation Theory: from skepticism to support
- From Bachelier to Data Science: A.I. and M.L.
- The Theory of Speculation: a timeless framework or outdated concept?
- Conclusions
- Bibliography
The Theory of Speculation
Louis Bachelier’s groundbreaking research in the early 20th century documented the similarities between random-number sequences and the time profiles of actual wheat and stock prices. His work introduced the concept of random walks in financial markets and provided a framework for understanding price changes. However, his strict application of the absolute Gaussian distribution faced opposition due to the limitations of common stocks, which cannot have negative prices. The concept of geometric Brownian motion, proposed by some researchers, addressed this limitation and further advanced the notion of efficient markets.
THÉORIE
DE LA
SPÉCULATION
— PAR M. L.
BACHELIER
The dissertation is famous for application of stochastic analysis in a Brownian Walk, predating Einstein by some five years (Thomson, 2016). Bachelier found out that prices fluctuates randomly and are statistically independent as well as identically distributed. His ideas challenged conventional wisdom and sparked a revolution in how we understand financial markets.
Bachelier’s thesis centered around the idea that price changes in financial markets follow a random walk. He argued that future price movements cannot be predicted based on past prices, as they are determined by a series of independent and unpredictable events. In the Theory of Speculation, this concept of a random walk challenged the prevailing belief that markets could be forecasted using deterministic models.
Bachelier’s Speculation Theory, defended on March 29, 1900, at the Sorbonne, laid the foundation for modern options pricing theory and stochastic analysis. Interestingly, Bachelier’s work remained relatively overlooked in the field of financial economics for several decades. It was only rediscovered by financial economists more than fifty years later. Once his ideas gained recognition, the field of option pricing rapidly advanced, culminating in the seminal work of Fischer Black, Myron Scholes, and Robert Merton in 1973. Bachelier’s pioneering efforts set the stage for subsequent developments that transformed the understanding and application of mathematical finance.
What is meant by Random Walks?
The concept of a random walk in finance is a way to describe the unpredictable nature of price movements in financial markets. It suggests that the future price of a financial asset, such as a stock or a currency, cannot be reliably predicted based on its past price movements.
To understand this concept, let’s imagine you’re taking a walk in a park. You start at a specific point and take a series of steps. In a random walk, each step you take is completely random and unrelated to the previous step.
You might take a step forward, then two steps backward, then three steps to the left, and so on. The direction and length of each step are unpredictable.
Now, let’s apply this metaphor to finance. In the context of financial markets, the price of an asset can be thought of as your position in the park. Each day or time period, the price can move up or down, just like taking a step forward or backward in the random walk. However, these price movements are influenced by various factors like market news, economic data, investor sentiment, and other unpredictable events: technically, they can follow a Brownian motion.
Bachelier's use of Brownian motion as a mathematical model for price changes was another groundbreaking aspect of his work. Brownian motion is a stochastic process characterized by random fluctuations, similar to the movement of particles in a fluid. Bachelier suggested that price changes could be modeled using Brownian motion, which provided a mathematical framework for understanding and analyzing market dynamics.
As a consequence, this concept suggests that there is no consistent pattern or trend that can be exploited to predict future price movements accurately. It implies that using historical price data alone is not sufficient to reliably forecast future prices, as each new price movement is independent and random.
From Bachelier’s stochasticity to alternative approaches
Stochastic analysis is a branch of mathematics that deals with processes that involve randomness, such as the movement of stock prices. In this sense, Bachelier has provided a framework for analyzing and predicting the behavior of these random processes.
While stochastic analysis can provide valuable insights, it’s important to note that it is not a crystal ball for price prediction, especially in case of option valuation. Financial markets are influenced by a multitude of factors, including economic conditions, investor sentiment, geopolitical events, and unexpected news. These factors introduce complexities and uncertainties that make it challenging to predict prices accurately.
An example? Think about the history of the French rentes (i.e. government debt) and the impact of political crises and the collapse of the French franc highlight the complexities of financial markets and the challenges in modeling their behavior.
In addition to stochastic analysis, there are other approaches and tools used in price prediction and financial forecasting. Some commonly employed methods include:
- Machine Learning and Data Science: With the advancements in AI and machine learning, these techniques are increasingly used to analyze large datasets and uncover patterns or relationships that may help predict future price movements. Machine learning algorithms can be trained on historical data to identify trends, correlations, and anomalies that may be indicative of future price behavior.
- Econometric Models: Econometric models use statistical techniques to analyze economic data and make predictions. These models incorporate various economic indicators, such as interest rates, inflation, GDP growth, and industry-specific data, to estimate the impact on asset prices. Econometric models aim to capture the relationships between these variables and asset prices.
- Sentiment Analysis: Sentiment analysis involves analyzing social media feeds, news articles, and other sources of information to gauge market sentiment and investor attitudes. By monitoring and quantifying positive or negative sentiment, it is believed that market sentiment can be used as a contrarian indicator to predict price movements.
- Event-Based Analysis: Event-based analysis involves studying the impact of specific events or announcements on asset prices. It focuses on identifying and evaluating the potential market reaction to events such as earnings releases, product launches, regulatory changes, or geopolitical developments.
It’s important to understand that no single tool or approach can guarantee accurate price predictions. Often, a combination of methods and a comprehensive understanding of market dynamics are necessary for making informed investment decisions.
Additionally, it’s crucial to exercise caution, consider multiple perspectives, and incorporate risk management strategies when utilizing any prediction tools in financial markets.
Bachelier’s Speculation Theory: from skepticism to support
As we’ve seen, Bachelier’s assumption implies that the probability of large price movements is quite low, and most price changes are relatively small. However, critics argue that real-world price fluctuations often deviate from the strict assumptions of Bachelier’s Theory of Speculation. Financial markets can experience extreme price swings, especially during periods of high volatility or market crises.
Another factor that impacted the validity of Bachelier’s theory is the efficient market hypothesis (EMH) by Samuelson (1965) and Fama (1963, 1965). The EMH states that financial markets are efficient, meaning that prices fully reflect all available information. According to the EMH, it is impossible to consistently achieve above-average returns through trading strategies based on past price patterns or other historical data. Bachelier’s random walk theory aligns with the EMH, as it implies that past prices do not contain any useful information for predicting future prices.
Milton Friedman, a distinguished economist, reacted adversely to Harry Markowitz’s portfolio optimization paradigm, showing resistance to post-Bachelier finance (Davis & Etheridge, 2006).
However, critics of the EMH argue that markets are not always perfectly efficient. They point to instances of market inefficiencies and anomalies that can be exploited for profit. Behavioral economists, in particular, emphasize that human biases and irrational behavior can lead to pricing distortions and deviations from the efficient market ideal. These behavioral models acknowledged that market participants do not always behave in a rational manner, providing opportunities for traders to profit from correcting aberrant pricing patterns.
In fact, Stochastic methods in finance were initially revolutionary and faced resistance from economists who focused on understanding the fundamentals of financial markets within an economic system. Once the Black-Scholes-Merton model has been officially recognized as a winning solution for option-pricing problem, Bachelier received the attention he deserves also. Indeed, the tools introduced by Bachelier – particularly his application of stochastic analysis and martingale assumption – had a significant impact on economists like Paul Samuelson, who recognized their usefulness in addressing the option pricing problem (Samuelson, 1965).
From Bachelier to Data Science: A.I. and M.L.
The revolution in Artificial Intelligence (AI), Machine Learning (ML), and in general data science has indeed opened up new possibilities for analyzing financial markets and making predictions. Researchers and practitioners are constantly exploring ways to incorporate past research, such as the works of Bachelier, Scholes, Markowitz, and others, into modern quantitative models and algorithms.
For instance, Bachelier’s strict text involved price changes subject to absolute Gaussian distribution, but limited-liability common stocks cannot have negative prices, leading to the suggestion of using geometric Brownian motion based on log-Gaussian distributions. Thus, for predicting geometric Brownian motion, today we can consider models such as recurrent neural networks (RNNs) or long short-term memory (LSTM) networks.
Moreover, machine learning algorithms can analyze large datasets to identify complex nonlinear relationships, detect hidden patterns, and make predictions based on historical market data. By incorporating various inputs such as market indicators, economic factors, news sentiment, and even alternative data sources, these algorithms can potentially improve the accuracy of price predictions and identify profitable trading opportunities.
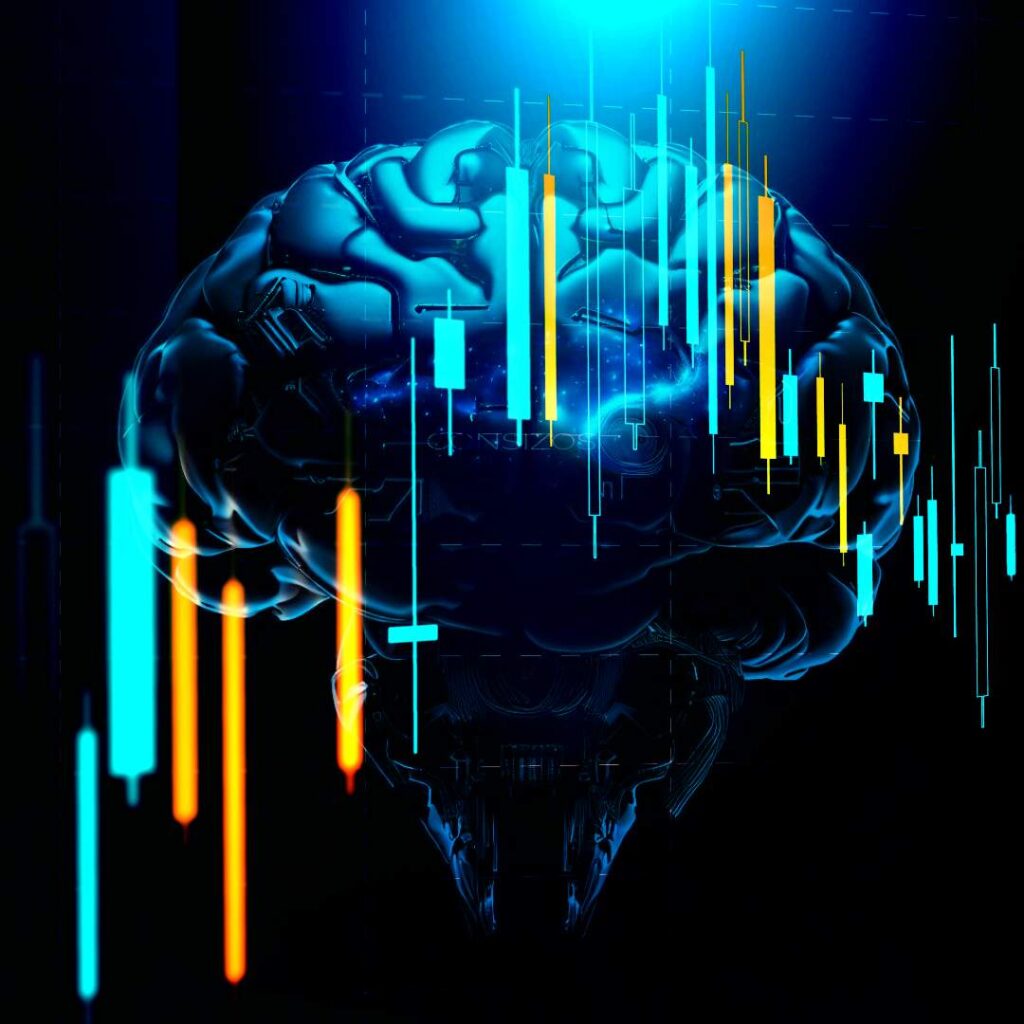
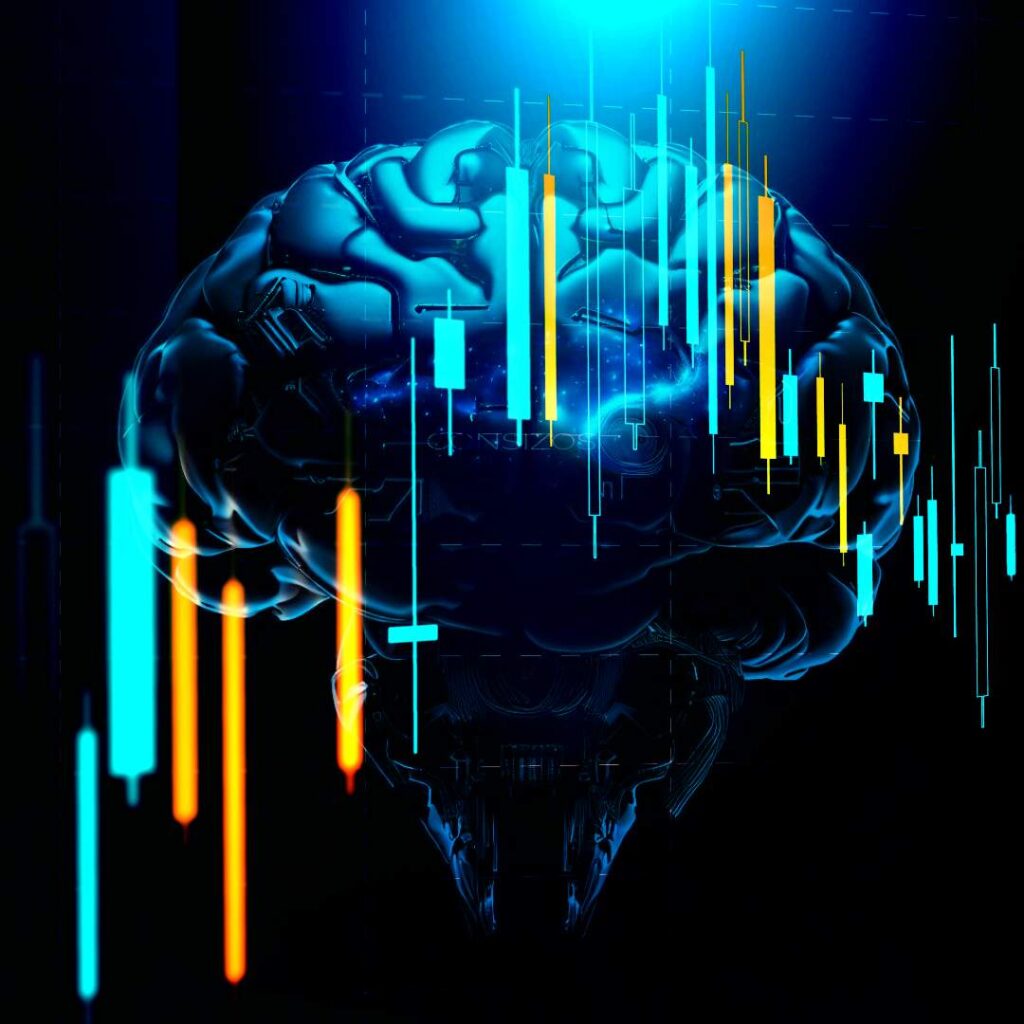
The Theory of Speculation: a timeless framework or outdated concept?
Louis Bachelier’s Speculation Theory laid the groundwork for modern mathematical finance and was a pioneering contribution to the field. While the specific formula he derived for option pricing was not identical to the later Black-Scholes-Merton model, Bachelier’s ideas and concepts were instrumental in shaping the development of option pricing theory.
It’s important to note that financial markets and our understanding of them have evolved significantly since Bachelier’s time. New models, theories, and empirical research have emerged, refining our understanding of market dynamics and pricing. The field of mathematical finance has grown in complexity and sophistication, incorporating advancements in stochastic analysis, econometrics, and computational methods.
Moreover, behavioral and human aspects are playing a crucial role, especially in times where investing is within everyone’s reach.
While Bachelier’s original formula may not be directly applicable to today’s financial markets, his insights into modeling randomness and uncertainty in asset prices are still relevant. The principles and concepts he introduced continue to underpin much of the modern quantitative finance framework.
Today, option pricing models have evolved considerably, incorporating factors such as interest rates, dividend yields, and market volatility to provide more accurate valuations. The Black-Scholes-Merton model, along with subsequent enhancements and variations, remains widely used both in theory (mostly with the assumptions about volatility, that can be predicted with certainty and non-existent arbitrage in the marketplace) and in practice (Merton, 1992). However, it’s important to recognize that no model can perfectly capture all aspects of real-world financial markets, and ongoing research continues to refine and expand our understanding.
Conclusions
Bachelier’s contributions to the theory of speculation and the development of stochastic analysis have had a lasting impact on modern finance. Despite initial resistance, his ideas paved the way for the efficient market hypothesis and the application of mathematics in understanding financial markets. From Bachelier’s work to the present day, financial mathematics has continued to evolve, offering new insights and tools for investors, economists, and mathematicians. The Theory of Speculation turned out to be a revolutionary model for those times, however it remains a model, a simplification of reality, as well as AI, machine learning, and data science models: they can enhance our understanding and predictive capabilities, but they should be viewed as tools that complement traditional financial analysis and expertise. Successful prediction of price fluctuations still requires a combination of domain knowledge, experience, and a critical evaluation of the model’s outputs.
Bibliography
MARK DAVIS & ALISON ETHERIDGE (2006). Louis Bachelier’s Theory of Speculation. The origin of modern finance. Princeton University Press: New Jersey.
PAUL SAMUELSON (1965). Rational theory of warrant pricing. Industrial Management Review 6:13–39.
ROBERT MERTON (1973). Theory of rational option pricing. Bell Journal of Economics and Management Science 4:141–183. (Chapter 8 of Merton (1992)).
THOMSON, IAN (2016). Option Pricing Model comparing Louis Bachelier with Black-Scholes Merton. 10.13140/RG.2.1.3896.7446.